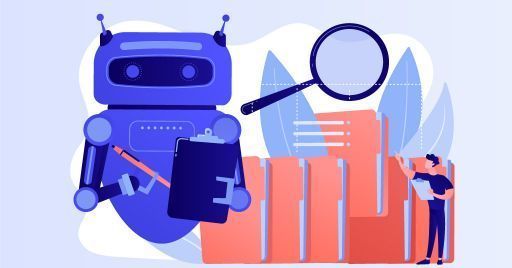
How AI-Driven Analytics is redefining eLearning
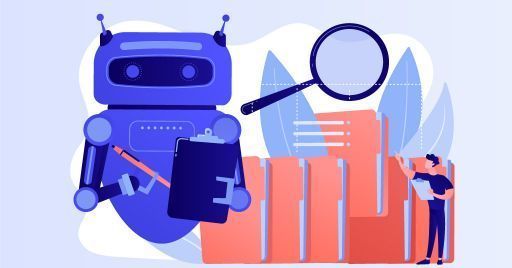
AI-driven data analytics is revolutionizing the world of education. Let’s explore how this transformation is taking place and the tools involved.
AI-driven analytics are invaluable for identifying areas of improvement in eLearning and bridging the gap between traditional teaching methods and the evolving needs of modern learners. By leveraging artificial intelligence, educators and institutions can redefine their eLearning strategies to create more personalized, data-driven, and impactful learning experiences.
The rise of ai-driven analytics in eLearning
AI-driven data analytics refers to the use of artificial intelligence (AI) technologies to analyze large amounts of data generated by eLearning platforms. This data includes everything from user engagement metrics to test results and even more complex behavioral patterns, such as students' preferred learning styles. Using sophisticated algorithms, AI can process this data to uncover insights often invisible to the human eye, enabling educators to make informed decisions on how to improve their eLearning offerings.
Several key factors drive the rise of AI in eLearning analytics:
- The data boom: with the proliferation of digital learning tools, the volume of data generated by students has grown exponentially. AI provides the means to analyze this data at scale, turning it into useful information.
- The need to improve outcomes: institutions face pressure to demonstrate the effectiveness of their eLearning programs. AI can help track and predict student success, allowing for timely interventions to improve outcomes.
- Enhancing personalization with AI: modern students expect personalized experiences that cater to their individual needs and preferences. AI-driven analytics can identify unique learning styles and tailor content accordingly.
Analytics for personalized learning
One of the most significant impacts of AI-driven data analytics in eLearning is the ability to offer highly personalized learning experiences. Traditional education often relies on a one-size-fits-all approach, but AI allows for a customized experience for each student. This adaptive learning approach ensures that every student receives the support needed to progress in their education.
Predictive analytics for proactive interventions
Another powerful application of AI-driven analytics in eLearning is predictive analytics, which involves using data to forecast future outcomes. In an educational context, this means predicting the likelihood of a student succeeding or failing in a course, identifying potential dropouts, and recognizing signs of disengagement to act quickly. For example, if an AI model predicts that a student is at risk of falling behind, teachers can intervene with additional resources, feedback, or individual support. This approach not only improves the student's chances of success but also increases retention rates.
Data-driven decision-making for educators
AI-driven analytics can also positively influence educators' decision-making when designing and refining eLearning strategies. By analyzing trends and patterns in student data, educators can identify the most effective content and teaching methods for their students and the gaps in the curriculum. For example, if the data shows that a significant portion of students struggles with a particular module, educators can revise the content, perhaps breaking it down into smaller, more digestible parts or incorporating multimedia elements to enhance understanding.
Overcoming challenges and ethical considerations
While AI-driven analytics offer tremendous potential, they also come with challenges and ethical considerations. One of the main concerns is data privacy. With the collection of large amounts of personal data, it is crucial to ensure that this information is handled securely and ethically. Institutions must implement robust data protection measures and be transparent with learners about how their data is being used.
Another challenge is the risk of over-reliance on AI. While it can provide valuable insights, it should not replace the human element in education. Educators play a crucial role in interpreting the data generated by AI and making nuanced decisions that consider the broader context of a student’s learning experience.
9 AI-driven technologies for eLearning
Here are some key technologies used in eLearning that rely on AI:
1. Machine Learning
Functionality: Machine Learning algorithms analyze large amounts of student data to identify patterns and make predictions. These systems adapt based on the data without being explicitly programmed for specific tasks.
Application in eLearning: Machine Learning is used to create adaptive learning systems that tailor content to individual students' needs, predict student performance, and recommend personalized learning paths.
2. Natural Language Processing (NLP)
Functionality: NLP enables computers to understand, interpret, and respond to human language. These algorithms process text or speech data, extract meaningful information, and can respond to students instantly.
Application in eLearning: NLP powers chatbots and virtual assistants that provide instant feedback and support. It also performs emotional analysis: the software can evaluate learner engagement and emotional responses to content.
3. Big Data Analytics
Functionality: Big Data Analytics involves processing and analyzing large datasets to uncover patterns, trends, and correlations that might be missed with smaller datasets.
Application in eLearning: this technology is useful for analyzing behaviors, tracking progress, and measuring the effectiveness of content across groups of students, providing insights to improve instructional design.
4. Artificial Neural Networks (ANNs)
Functionality: ANNs are computing systems inspired by the network of neurons in the human brain. They can learn from data, recognize patterns, and make complex decisions.
Application in eLearning: ANNs are complex systems used in adaptive learning technologies to predict outcomes, such as student performance, and to personalize educational materials based on these predictions.
5. Data Mining
Functionality: data mining is the process of discovering patterns and relationships in large datasets using statistics and Machine Learning techniques.
Application in eLearning: data mining helps identify key factors that influence outcomes, such as the most effective types of content, learning behaviors that predict success, and early signs of student disengagement.
6. Recommendation systems
Functionality: recommendation systems suggest content, courses, or learning paths to users based on their past behavior, preferences, and performance.
Application in eLearning: they personalize the learning experience by recommending relevant resources, tasks, or courses tailored to individual learning needs.
7. Predictive Analytics
Functionality: predictive analytics uses data, statistical algorithms, and Machine Learning techniques to forecast future outcomes.
Application in eLearning: it predicts student success, identifies potential dropouts, and suggests interventions before problems arise.
8. Blockchain technology
Functionality: blockchain guarantees data security, transparency, and immutability.
Application in eLearning: blockchain can be used to securely store and verify educational credentials, track learning progress across different platforms, and ensure the integrity of assessment data.
Did you like this article? Sign up for the newsletter and receive weekly news!
Subscribe to NewsletterComments:
No comments are in yet. You be the first to comment on this article!