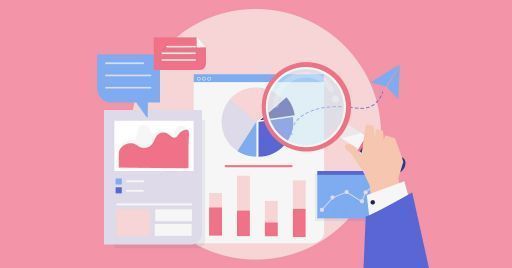
Data analysis in eLearning: a beginner's guide
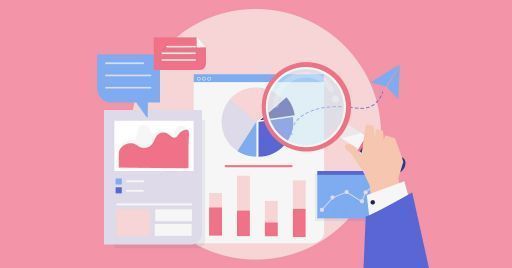
In this article we explore the process of data analysis in eLearning, offering a simple guide for those new to this world.
In today's world, the ability to analyze and interpret data is becoming increasingly crucial in all fields. From business and healthcare to education and beyond, data analytics enables individuals and organizations to make informed decisions, solve complex problems, and achieve meaningful results.
However, for those new to this activity, the realm of data analysis can often seem daunting and complex. In this article we want to demystify the process of data analysis, providing a beginner's guide that will help you use this valuable skill with confidence and make the most of it in online learning.
What is data analysis
Data analytics is the process of examining, filtering, transforming, and interpreting data to uncover meaningful preferences, insights, trends, and patterns. Whether it is numerical data, text, images or other forms of information, the goal of data analysis is to extract key information that can be used in decision making.
In the case of eLearning, data analysis is essential to understand student behavior, improve the effectiveness of courses and optimize educational design.
Data analysis applied to eLearning
As we have seen, data is crucial to improving your training offering. However, managing and protecting them is a responsibility that should not be underestimated. Read also “How to manage student data”.
But what are the main techniques for analyzing learning data?
Data collection: the first step of data analysis is the collection of relevant data from various sources. This can be surveys, databases, sensors, social media or other sources, depending on your specific needs and objectives.
Data cleaning and preparation: raw data is often messy and incomplete, requiring cleaning and preprocessing before analysis. These include tasks such as removing duplicates, managing missing values, and standardizing formats to ensure accuracy and consistency.
Exploratory Data Analysis (EDA): EDA involves the visual exploration and synthesis of data to understand its underlying structure and relationships. Techniques such as histograms, scatter plots, and summary statistics can help uncover patterns and anomalies.
Statistical analysis: Statistical methods allow you to quantify relationships and test hypotheses within your data.
To simplify things we can say that there are three types of analysis useful in the field of eLearning: descriptive, predictive and prescriptive analysis:
- descriptive analysis: descriptive analysis focuses on summarizing past data to understand what happened. Provides insights into student behavior and performance trends.
- predictive analytics: predictive analytics uses historical data to predict future trends and results. In eLearning, predictive analytics can help identify at-risk students or anticipate performance gaps.
- prescriptive analytics: prescriptive analytics goes beyond predicting future results and suggests actions to take to achieve the desired results. Help recommend personalized learning interventions based on individual student needs.
Machine learning: machine learning algorithms can be used to build predictive models and discover complex patterns in data. Techniques such as classification, clustering and regression can help automate decision-making processes and identify trends.
Tools and technologies for data analysis
A wide range of tools and technologies are available to facilitate data analysis, from traditional spreadsheets to more advanced programming languages and frameworks (in the latter case developer knowledge is required). Additionally, so-called data visualization tools can help effectively communicate insights through interactive charts and dashboards.
The right data analytics technology depends on the needs and size of your business. You can start small by collecting data from questionnaires in case you are dealing with a small number of people; but if you want to collect and analyze data from many people it will be necessary to rely on more advanced technologies.
Best practices for effective data analysis
Below we see step by step the practices necessary to implement correct data analysis.
- Define goals and metrics
- The first step is to identify the key objectives of your eLearning program. In other words, you will need to ask yourself what you want to find out from this data.
- Define measurable metrics aligned with these objectives that allow you to obtain data that answers the question you ask yourself (for example, if you are trying to understand the level of information retention of your course, try to collect data on completion rates, levels of engagement and evaluation scores).
- Collect data
- Use learning management systems (LMS) to collect data on learners' activities, progress and performance.
- Incorporate tracking tools like xAPI (Experience API) to capture more granular data, such as media interactions or simulations.
- Implement surveys, quizzes, and assessments to gather qualitative and quantitative feedback from learners.
- Filter and organize your data
- Eliminate duplicates, inconsistencies and irrelevant data points.
- Standardize data formats and labels to facilitate analysis.
- Organize data into relevant categories or segments (e.g. demographics, course modules, etc.).
- Analyze the data
- Use statistical methods and visualization techniques to explore patterns and trends in the data.
- Calculate basic descriptive statistics (mean, median, mode, standard deviation) to understand trends and variability.
- Conduct comparative analyzes between different groups of students or courses to identify correlations.
- Use data visualization tools such as graphs, charts, and heat maps to present results clearly.
- Interpret the results
- Draw insights from data analysis in relation to defined objectives.
- Identify areas of strength and improvement in the eLearning program.
- Explore the factors that influence learner engagement, retention and performance.
- Consider qualitative feedback alongside quantitative metrics for a complete understanding.
- Implement the changes
- Based on the insights gained, make data-driven decisions to improve the eLearning experience.
- Modify course content, delivery methods, or assessment strategies to address identified issues.
- Experiment with personalized learning paths or adaptive learning techniques to better meet individual student needs.
- Constantly monitor the impact of changes.
- Evaluate effectiveness
- Measure the effectiveness of interventions or improvements implemented based on data-driven decisions.
- Track changes in key metrics over time to evaluate progress.
- Ensure there is a constant feedback loop from students and instructors to gauge satisfaction and perceived improvements.
- Ensure data privacy and security
- Adhere to privacy policies and best practices for managing learner data.
- Anonymize data when necessary to protect learner privacy.
- Implement robust security measures to safeguard data from unauthorized access or breaches.
- Continuously improve
- Treat data analysis as an ongoing process rather than a one-off activity.
- Regularly review and update data collection methods, metrics, and analysis techniques to adapt to evolving needs and technologies.
- Promote a culture that teaches and explains the process of data analysis and evidence-based decision making among eLearning stakeholders.
Did you like this article? Sign up for the newsletter and receive weekly news!
Subscribe to NewsletterComments:
No comments are in yet. You be the first to comment on this article!